Published:
May 2004
Proceedings:
Proceedings of the Seventeenth International Florida Artificial Intelligence Research Society Conference (FLAIRS 2004)
Volume
Issue:
Proceedings of the Seventeenth International Florida Artificial Intelligence Research Society Conference (FLAIRS 2004)
Track:
All Papers
Downloads:
Abstract:
Thenecessity for improved players and opponents in firstperson entertainment-based real-time artificial environments has inspired our research into artificial game players. We employed the approach of creating agent-based players, one using simple reflex-action based components and another based on a back propagation neural network, to interact in a modified Quake II environment. Our evaluation of these agents utilizes two metrics, a statistical measure and graph similarity based on clustering from player performance traces against interactive feature points in the environments—both comparing the agents to a sample of 20 human players. The reflex-based agent was able to complete 29 of 100 test levels with 73.1% within statistical humanperformance levels and 15.4% similar to the human players, while the BPNN-based agent was unable to complete any levels. These results, including some additional findings that reveal the potential for a combinational approach, are inspiring an architectural vision of a multi-agent system to provide an advanced artificial intelligence engine for the embodiment of advanced artificial characters in gaming and simulation domains.
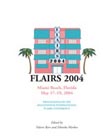
FLAIRS
Proceedings of the Seventeenth International Florida Artificial Intelligence Research Society Conference (FLAIRS 2004)
ISBN 978-1-57735-201-3
Published by The AAAI Press, Menlo Park, California.