Published:
May 2004
Proceedings:
Proceedings of the Seventeenth International Florida Artificial Intelligence Research Society Conference (FLAIRS 2004)
Volume
Issue:
Proceedings of the Seventeenth International Florida Artificial Intelligence Research Society Conference (FLAIRS 2004)
Track:
All Papers
Downloads:
Abstract:
The normal operation of a device can be characterized in different temporal states. To identify these states, we introduce a clustering algorithm called Gecko that can determine a reasonable number of clusters using our proposed L method. We then use the RIPPER classification algorithm to describe these states in logical rules. Finally, transitional logic between the states is added to create a finite state automaton. Our empirical results, on data obtained from the NASA shuttle program, indicate that the Gecko clustering algorithm is comparable to a human expert in identifying states and our overall system can track normal behavior and detect anomalies.
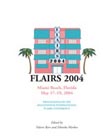
FLAIRS
Proceedings of the Seventeenth International Florida Artificial Intelligence Research Society Conference (FLAIRS 2004)
ISBN 978-1-57735-201-3
Published by The AAAI Press, Menlo Park, California.