Published:
May 2004
Proceedings:
Proceedings of the Seventeenth International Florida Artificial Intelligence Research Society Conference (FLAIRS 2004)
Volume
Issue:
Proceedings of the Seventeenth International Florida Artificial Intelligence Research Society Conference (FLAIRS 2004)
Track:
All Papers
Downloads:
Abstract:
When designing any type of fuzzy rule based system, considerable effort is placed in identifying the correct number of fuzzy sets and the fine tuning of the corresponding membership functions. Once a rule base has been formulated a fuzzy inference strategy must be applied in order to combine grades of membership. Considerable time and effort is spent trying to determine the number of fuzzy sets for a given system while substantially less time is invested in obtaining the most suitable inference strategy. This paper investigates a number of theoretical proven fuzzy inference strategies in order to assess the impact of these strategies on the performance of a fuzzy rule based classifier system. A fuzzy inference framework is proposed, which allows the investigation of five pure theoretical fuzzy inference operators in two real world applications. An additional two novel fuzzy-neural strategies are proposed and a comparative study is undertaken. The results show that the selection of the most suitable inference strategy for a given domain can lead to a significant improvement in performance.
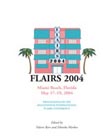
FLAIRS
Proceedings of the Seventeenth International Florida Artificial Intelligence Research Society Conference (FLAIRS 2004)
ISBN 978-1-57735-201-3
Published by The AAAI Press, Menlo Park, California.